In the vast world of artificial intelligence, data access plays a pivotal role in the success and development of AI systems. For AI to be effective, it relies heavily on data to improve its accuracy, speed, and decision-making capabilities. As AI continues to evolve, particularly with large language models (LLMs), the need for high-quality, reliable, and diverse data has become essential.
Unlocking data access for AI is not just about feeding systems with more data—it’s about ensuring that this data is trustworthy and accurate. By prioritizing data transparency and reliability, we can enhance AI performance, build trust with users, and open new opportunities for innovation. The future of AI depends on how effectively we manage and provide access to the data it requires.
Table of Contents
The Importance of Data Access for AI
Enhancing AI Accuracy
Data access for AI is essential for improving the accuracy of AI models. High-quality, diverse, and extensive datasets enable AI systems to learn more effectively, reducing errors and enhancing performance. For instance, large language models like OpenAI‘s GPT-4 require vast amounts of text data to understand and generate human-like responses accurately. The more data these models have, the better they can perform.
Addressing LLM Bias
Bias in AI is a significant concern, especially with LLMs. Biases can emerge from unbalanced training data, leading to unfair or inaccurate outcomes. However, by ensuring comprehensive data access, AI developers can include diverse datasets that help mitigate bias. Consequently, this approach promotes fairer, more equitable AI systems that can serve a broader range of users effectively.
Facilitating Responsible AI Development
Responsible AI development hinges on transparent and ethical data usage. Access to diverse and representative datasets allows developers to train AI systems that are not only accurate but also ethically sound. This practice involves scrutinizing data sources, ensuring consent, and respecting privacy, which collectively build user trust and acceptance.
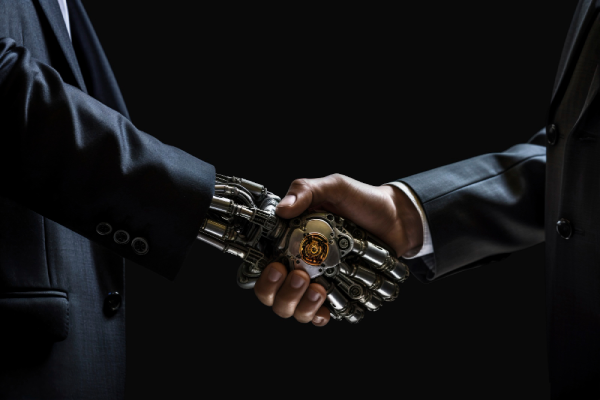
Publisher Partnerships with AI Companies
Strengthening Data Sources
Collaborations between publishers and AI companies are pivotal in enhancing data access. Publishers possess vast amounts of high-quality, verified content that can significantly improve AI training datasets. These partnerships ensure that AI systems have access to reliable and up-to-date information, boosting their accuracy and reliability.
Enhancing Fact-Checking AI
Fact-checking is a critical application of AI, especially in combating misinformation. Partnerships with reputable publishers provide AI systems with access to verified facts and credible sources. This integration enhances the AI’s ability to verify information and deliver trustworthy outputs, thereby increasing user trust in AI-driven solutions.
Future of AI through Collaboration
The future of AI lies in collaborative efforts among various stakeholders, including publishers, tech companies, and regulatory bodies. By working together, these entities can create robust data ecosystems that fuel the next generation of AI advancements. Consequently, these collaborations will drive innovations, ensuring AI systems are accurate, trustworthy, and aligned with societal values.
Trustworthy AI
In the rapidly evolving world of AI, gaining and maintaining user trust is essential for ensuring long-term success and broad acceptance. Trustworthy AI isn’t just about flawless functionality; it’s about transparency, accountability, and ethical responsibility. Users are more likely to embrace AI systems that lay bare their decision-making processes and the sources of the data they rely on. When an AI system clearly communicates how it has been trained and demonstrates its commitment to fairness, it strengthens the bond with its audience. After all, trust is built on consistency, and AI systems that adhere to ethical guidelines, prioritize user privacy, and ensure data security create a safer, more engaging experience for everyone involved.
Building User Trust
Trustworthy AI is built on transparency, reliability, and ethical considerations. Moreover, users are more likely to trust AI systems that demonstrate transparency in their data sources and decision-making processes. Additionally, clear communication about how AI systems are trained and the types of data they use is essential for building user confidence.
Responsible AI Practices
Implementing responsible AI practices involves continuous monitoring and evaluation of AI systems to ensure they operate ethically and without bias. Additionally, this includes regular audits of training data, transparent reporting of AI decision-making processes, and implementing safeguards to protect user privacy and data security.
AI Training Data
Importance of High-Quality Data
In the ever-evolving landscape of AI, high-quality data serves as the bedrock for creating robust and effective models. To truly harness the power of AI, the data used in its training must not only be accurate and relevant but also mirror the complexities and nuances of the real world. When data is skewed or lacks depth, it compromises the system’s ability to make reliable predictions, resulting in unpredictable or even faulty outcomes. By prioritizing data that is both comprehensive and diverse, we enable AI to evolve in a way that is not only precise but also trustworthy, ensuring its impact remains both meaningful and transformative across industries.
Strategies for Data Collection
Effective data collection strategies involve sourcing data from diverse and credible sources. This includes leveraging data from publisher partnerships, public databases, and user-generated content, ensuring a broad and representative dataset. Ethical considerations, such as obtaining user consent and respecting privacy, are paramount in these strategies.
Addressing Data Gaps
Identifying and addressing data gaps is not just a crucial step—it’s the backbone of refining AI systems to their full potential. By consistently updating training datasets with fresh, relevant information, and meticulously correcting any biases or inaccuracies, we empower AI to evolve with precision. Bridging these gaps allows AI to deliver results that aren’t just accurate, but reliable and trustworthy. As the field of AI continues to expand, ensuring diverse, high-quality data access will be the key to unlocking smarter, more effective AI systems that can adapt and make decisions with confidence.
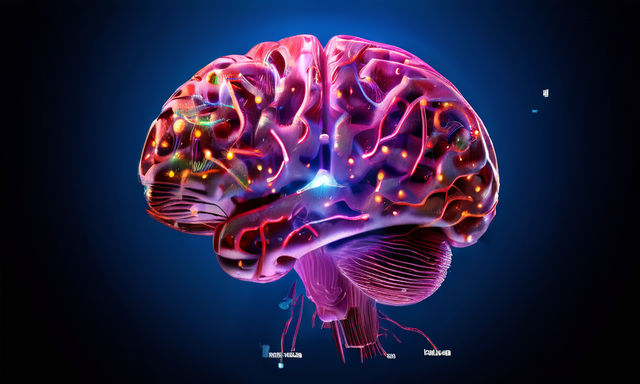
Large Language Models (LLMs)
Advancements in LLMs
Large Language Models (LLMs) have revolutionized natural language processing, enabling AI systems to understand and generate human-like text with remarkable accuracy. These advancements have transformed industries, from customer service to healthcare. However, the true potential of LLMs relies heavily on access to vast, diverse datasets. Data access for AI is crucial, as high-quality data fuels LLMs’ ability to perform effectively. Without it, these models would be limited, hindering innovation and progress. Thus, reliable data is the key to unlocking the full power of LLMs.
Challenges and Solutions
Training LLMs poses several challenges, such as managing vast amounts of data, addressing biases, and ensuring data quality. Resolving these issues entails employing advanced data management techniques, implementing bias mitigation strategies, and collaborating with diverse data sources to ensure the creation of comprehensive and accurate training datasets.
Fact-Checking AI
Role in Combatting Misinformation
In the fight against misinformation, AI-driven fact-checking tools are emerging as powerful allies. With the ability to sift through massive datasets and deploy sophisticated algorithms, these tools work tirelessly to validate the truth, ensuring users receive accurate, reliable content. In a world where misinformation spreads faster than ever, these AI tools serve as gatekeepers of truth, making their role more crucial than ever before.
Enhancing Accuracy with Data Access
For AI to truly excel in its mission to combat misinformation, it requires access to a rich variety of credible data sources. By integrating verified information from trusted publishers, AI systems can elevate their fact-checking capabilities, offering even more precise and dependable results. This infusion of high-quality data doesn’t just enhance the accuracy of fact-checking—it cultivates a culture of trust, ensuring that the digital space remains as factual and reliable as possible.
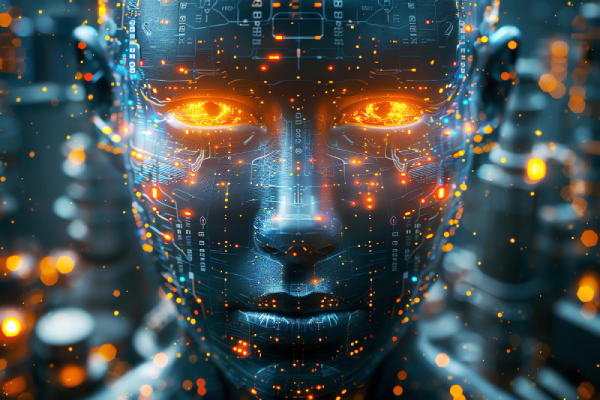
Future of AI
Innovations Driven by Data Access
The future of AI relies heavily on advancements in data access and management. As AI evolves, the demand for diverse, high-quality datasets continues to rise. Efficient data collection, storage, and processing will be key drivers of future breakthroughs. These innovations will unlock AI’s potential and reshape industries across the globe. Ultimately, data access will power the next wave of AI advancements, transforming how we interact with technology.
Ethical and Regulatory Considerations
Ethical and regulatory considerations will play a significant role in shaping the future of AI. Moreover, ensuring responsible data usage, protecting user privacy, and implementing transparent AI practices are essential for building public trust and acceptance. Additionally, collaborative efforts between tech companies, regulators, and other stakeholders will be crucial in navigating these challenges and fostering a sustainable and ethical AI ecosystem.
Conclusion
Unlocking data access for AI is essential for boosting accuracy and trust in AI systems. By leveraging high-quality, diverse datasets and fostering collaborations with reputable publishers, AI developers can create more reliable and trustworthy AI solutions. Additionally, addressing biases, implementing responsible AI practices, and continuously innovating in data management will ensure that AI systems are accurate, ethical, and aligned with societal values. Furthermore, as we move forward, the importance of data access for AI will only grow, driving advancements and shaping the future of artificial intelligence.