Introduction to Image Labeling with Generators
In today’s digital era, using image generator how do you label images has become a common practice across various industries. However, the effectiveness of these tools largely depends on how well users label the images. Proper image labeling is crucial for organization, retrieval, and accurate machine learning outcomes. Without precise labels, the generated images may not serve their intended purpose. This introduction to image labeling with generators will explore essential techniques and best practices to ensure users tag their images accurately and efficiently. Effective image labeling enhances the overall quality and usability of AI-generated content.
What Are Image Generators And using image generator how do you label images?
Image generators are advanced tools that use artificial intelligence to create images from textual descriptions. Various fields such as marketing, design, and entertainment widely use them. By generating realistic images, these tools save time and resources, allowing creators to focus on other tasks. They rely on machine learning models trained on vast datasets to produce accurate and high-quality images. Understanding how to label these generated images effectively is crucial for optimizing their usability and ensuring they meet the desired standards.
Challenges in using image generator how do you label images
Labeling generated images presents several challenges that must be addressed to ensure high-quality data. Handling large volumes of data and maintaining consistency and accuracy are two primary obstacles. These challenges can impact the effectiveness of machine learning models if not properly managed. Therefore, we will explore these challenges and discuss strategies to overcome them. Understanding these hurdles is essential for anyone looking to improve their image labeling process and achieve better results with AI-generated content.
Handling Large Volumes of Data
One significant challenge in image labeling is managing the sheer volume of data. ,Using image generator how do you label images often results in a large number of images that need accurate labeling. To handle this effectively, it is essential to implement efficient data management practices. Moreover, automated image labeling tools can help streamline the process, reducing the time and effort required. Additionally, organizing images into manageable batches can make the task less overwhelming and more systematic.
Ensuring Consistency and Accuracy
Maintaining consistency and accuracy in labeling is crucial for high-quality data. Inconsistent labels can lead to poor machine learning outcomes and unreliable models. To ensure consistency, establish clear labeling guidelines and provide training for labelers. Furthermore, regular audits and quality checks can help identify and correct any discrepancies. By focusing on accuracy and consistency, you can improve the reliability and performance of your AI-generated images.
Tools and Software for Image generator to label images
Using the right tools and software can significantly enhance the image labeling process. Various tools are available that offer features like automated suggestions and collaborative labeling. For example, Labelbox, SuperAnnotate, and RectLabel are popular choices that cater to different needs and skill levels. These tools help streamline the process, ensuring that labels are applied accurately and efficiently. Ultimately, choosing the right tool depends on your specific requirements and the scale of your labeling project.
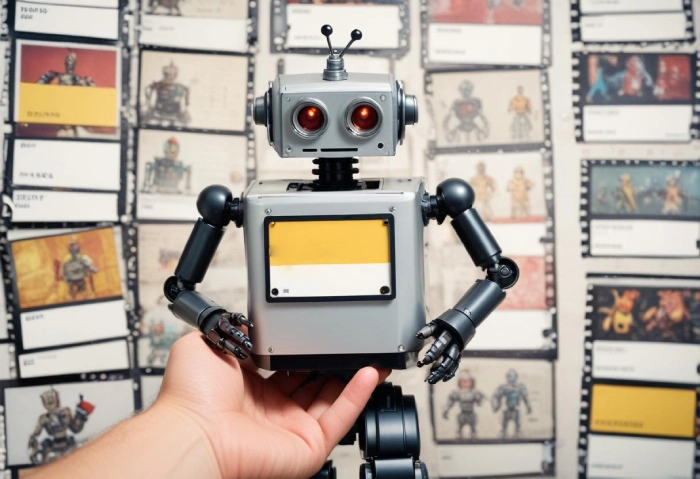
Best Practices for Image generator to label images
Implementing best practices in image labeling ensures high-quality, reliable data. Establishing clear labeling guidelines and ensuring quality control are key components of this process. Additionally, these practices help maintain consistency and accuracy, crucial for effective machine learning models. In this section, we will delve into these best practices and provide actionable tips to optimize your image labeling efforts.
Establishing Clear Labeling Guidelines
Clear labeling guidelines are the foundation of effective image labeling. These guidelines should define what each label represents and how to apply them. Consequently, consistency is achieved when all labelers follow the same rules, reducing the risk of errors. Moreover, providing detailed instructions and examples can help labelers understand and implement the guidelines correctly. Regularly updating these guidelines ensures they remain relevant and effective.
Ensuring Quality Control in Labeling
Quality control is essential for maintaining high standards in image labeling. Regular audits and reviews of labeled images help identify and correct any inaccuracies. Furthermore, implementing a system for quality checks, such as peer reviews or automated validation tools, can significantly improve the accuracy of your labels. By prioritizing quality control, you can ensure that your labeled images meet the required standards for effective machine learning.
Techniques for Efficient Image generator to label images
Efficiency is key when dealing with large datasets. Adopting effective techniques can streamline the labeling process and improve productivity. Batch labeling strategies and utilizing AI for assisted labeling are two methods that can enhance efficiency. Therefore, these techniques help manage large volumes of data while maintaining accuracy and consistency. In this section, we will explore these strategies in detail.
Batch Labeling Strategies
Batch labeling involves grouping similar images and labeling them together. This approach saves time and ensures consistency across related images. By focusing on one type of image at a time, labelers can work more efficiently and reduce the cognitive load. Additionally, batch labeling allows for easier quality control, as similar images can be reviewed together for accuracy.
Utilizing AI for Assisted Labeling
AI-assisted labeling leverages machine learning to automate part of the labeling process. Tools using AI can suggest labels based on learned patterns, which human labelers can then review and adjust. Consequently, this hybrid approach combines the speed of automation with the accuracy of human oversight. Utilizing AI for assisted labeling can significantly reduce the time and effort required, especially when dealing with large datasets.
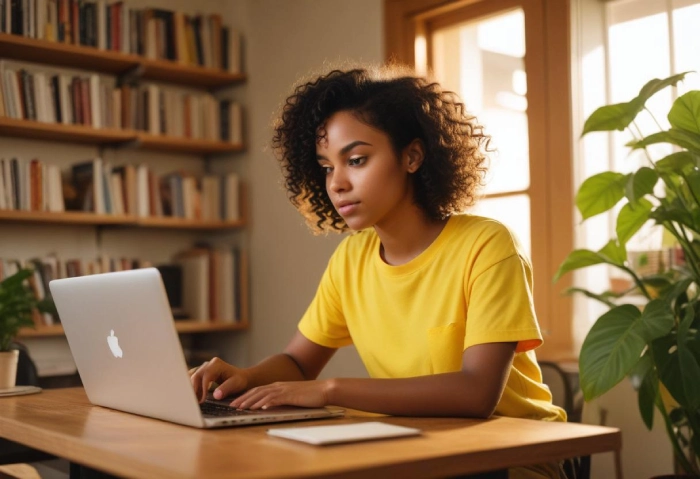
Leveraging Open Source Tools for Image Labeling
Open source tools offer flexible and cost-effective solutions for image labeling. These tools can be customized to meet specific needs, making them a popular choice for many organizations. In this section, we will discuss some popular open source labeling tools and how to customize them for your projects. Leveraging these tools can enhance your labeling process without significant financial investment.
Popular Open Source Labeling Tools
Several open source tools are available for image labeling, each offering unique features and capabilities. For example, tools like LabelImg, CVAT (Computer Vision Annotation Tool), and VGG Image Annotator (VIA) are widely used in the industry. These tools provide robust functionality for various labeling tasks and are supported by active communities. Therefore, exploring these options can help you find the right tool for your specific needs.
Customizing Open Source Solutions
Customizing open source tools allows you to tailor them to your specific requirements. This flexibility is one of the main advantages of open source software. Consequently, you can modify the code to add new features, improve performance, or integrate with other systems. By customizing open source solutions, you can create a labeling tool that perfectly fits your workflow and enhances your productivity.
Image Labeling in the Context of Big Data
In the era of big data, image labeling has become a crucial task. Managing and labeling large datasets efficiently is essential for leveraging big data technologies. These technologies help handle vast volumes of images, ensuring that each one is accurately labeled. Effective management practices and advanced tools streamline the labeling process, making it more manageable and less time-consuming. By incorporating big data technologies, organizations can improve the quality and speed of their image labeling efforts.
Managing and Labeling Large Datasets
Managing and labeling large datasets presents a significant challenge due to the sheer volume of images involved. Implementing scalable data management systems is crucial for organizing and processing these images effectively. Tools that support batch processing and automation can significantly ease this task. Additionally, systematic approaches, such as tagging images in batches, help maintain consistency and accuracy across extensive datasets. These strategies enhance the overall efficiency of the labeling process.
Leveraging Big Data Technologies
Big data technologies offer advanced solutions for handling extensive image datasets. These technologies enable the efficient storage, processing, and analysis of large volumes of data. Utilizing cloud-based platforms and distributed computing systems can greatly enhance the scalability of image labeling operations. Moreover, integrating big data tools with automated labeling systems helps streamline the workflow, ensuring that data is processed quickly and accurately. Leveraging these technologies improves both the speed and precision of image labeling.
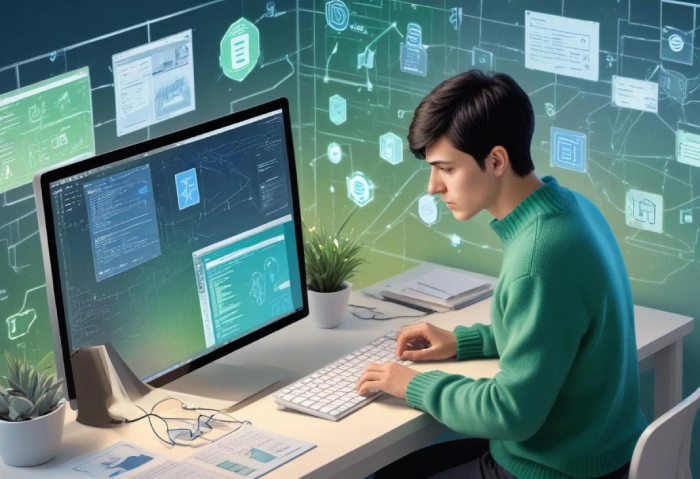
Leveraging AI to Improve Labeling Accuracy
Artificial Intelligence (AI) plays a vital role in enhancing labeling accuracy. AI algorithms can analyze images and suggest labels based on learned patterns and features. This assistance not only speeds up the labeling process but also improves the consistency of labels applied. By integrating AI with existing labeling systems, organizations can reduce human error and achieve more reliable results. Consequently, leveraging AI ensures that labeled data is of high quality, benefiting machine learning models and AI applications.
Assessing the Impact of Labels on AI Performance
The accuracy of labels directly impacts the performance of AI systems. Well-labeled data enhances the training and efficacy of machine learning models, leading to better predictions and outcomes. Evaluating how label quality affects AI performance helps identify areas for improvement. By analyzing the impact of labeling accuracy on model performance, organizations can refine their labeling practices and achieve more reliable results. Assessing this impact is crucial for optimizing AI systems and ensuring their effectiveness.
Future Directions in Image Labeling Research
The field of image labeling is continuously evolving, with ongoing research focused on improving labeling techniques. Future directions include developing more sophisticated AI algorithms for automated labeling and exploring new methods for managing large datasets. Additionally, research into integrating advanced technologies, such as deep learning and neural networks, promises to enhance labeling accuracy and efficiency. Staying updated with these advancements helps organizations adopt cutting-edge practices and stay ahead in the field of image labeling.
Conclusion
using image generator how do you label images, effective image labeling is crucial for harnessing the full potential of AI-generated images and big data technologies. By addressing challenges such as managing large datasets and leveraging advanced tools, organizations can improve labeling accuracy and efficiency. Embracing AI and staying informed about future research directions will further enhance labeling practices. Ultimately, accurate and efficient image labeling contributes significantly to the success of machine learning models and AI applications, ensuring high-quality results and effective data utilization.